Revolutionize your business strategy with AI-powered innovation consulting. Unlock your company's full potential and stay ahead of the competition. (Get started for free)
7 Ways AI-Enhanced Self-Service BI Tools Are Transforming Data Analysis in 2025
7 Ways AI-Enhanced Self-Service BI Tools Are Transforming Data Analysis in 2025 - Natural Language Data Queries Replace Complex SQL Commands in Power BI 2025
Power BI is moving towards a system in 2025 where users will increasingly be able to ask questions about data in everyday language, significantly diminishing the need for writing complicated SQL queries. This shift, powered by artificial intelligence, means anyone can get quick answers and data visualizations by simply typing questions in plain English. The Q&A function, conveniently located in dashboards, is intended to change the way people interact with data, providing instant results when asking questions about sales, profits and other data. This tool shows its answers in real-time, highlighting the growing importance of natural language technology in modern business data tools. As these easy-to-use natural language features spread, they have the potential to make data analysis a lot more accessible to a wider range of people, which has its own limitations too.
Power BI is integrating natural language interfaces, allowing users to phrase questions in ordinary language instead of using complex SQL queries; this makes data insights accessible to those without specific coding skills. Rather than needing a precisely constructed SQL statement, the system can interpret what a user means, adapting to various wordings. These systems are powered by machine learning that refines accuracy as it learns from user inputs. By 2025, these features should intelligently interpret incomplete questions and offer solutions, something databases using SQL are not able to do. Security measures are designed to apply data rules, ensuring confidential information is only accessible to those authorized while maintaining ease of use. The system remembers previous exchanges allowing for follow-up questions and more conversational data interactions unlike SQL's static nature. Multi-language capabilities are also part of the development allowing international users to query data without the need of english or coding. Domain-specific jargon can sometimes cause problems, therefore training and technological enhancement are required to avoid misunderstandings. Increased use of natural language implies less importance to SQL knowledge for new data analysts, and raises questions on the position of SQL in the future of data management. As natural language processing grows, the capability to visualize data straight from user questions becomes expected, letting them visualize data without complicated set-ups and in a very straight forward manner.
7 Ways AI-Enhanced Self-Service BI Tools Are Transforming Data Analysis in 2025 - Automated Dashboard Creation Cuts Report Building Time From Days to Minutes
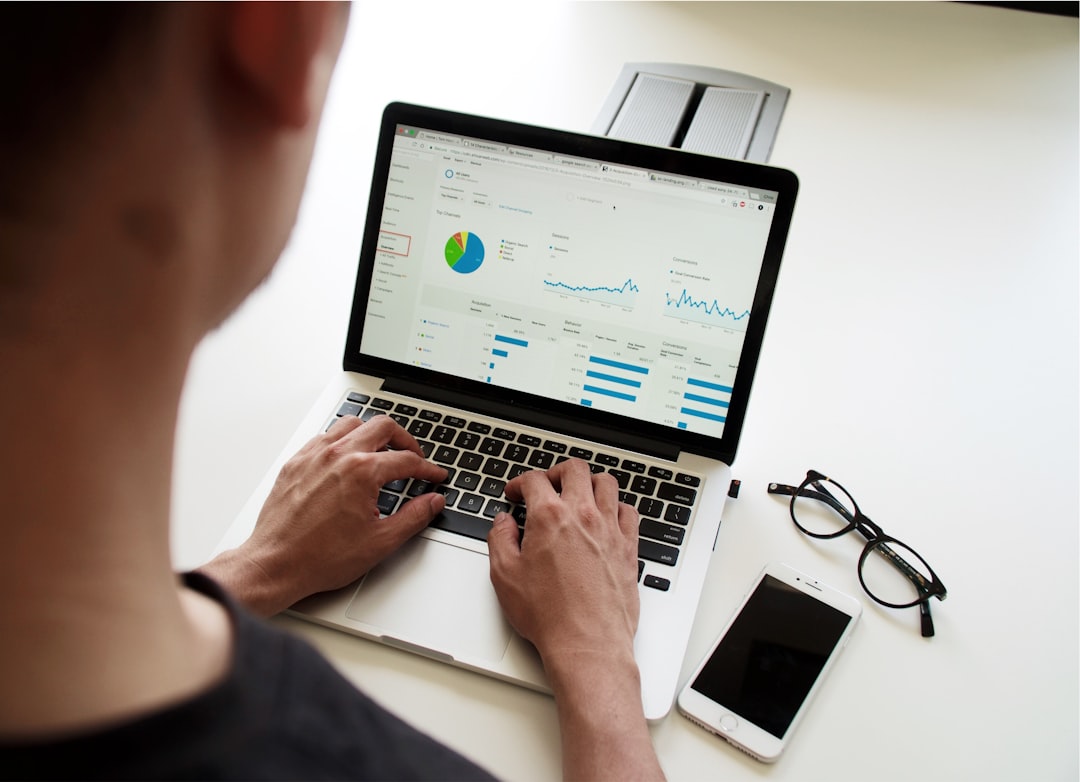
Automated dashboard creation is changing how people work with data, slashing the time it takes to make reports from days to just minutes. AI-powered tools now let users build their own dashboards without needing any coding expertise. Software like Polymer and Ajelix BI use AI to take raw data and turn it into visuals, letting users get quick understandings. These dashboards offer real-time analysis and interactive parts to improve both team transparency and decision-making. It represents a considerable change in how businesses are viewing and using data in 2025.
Automated dashboard generation uses pre-built designs which change automatically depending on data, this removes repeated design work which usually takes up valuable customization time. Because dashboards are made very quickly, in just a few minutes, organizations report saving about 70% of their time spent doing reports, enabling staff to focus on what the data is saying rather than how it looks. Complex mathematical rules can find patterns in data instantly, this processing speed is far faster than people could do and also helps reduce human error during data merging. Automated dashboards might even predict the future by spotting trends using past data, without users needing any expertise in statistics, therefore making complicated analysis tools available to everyone. Furthermore, automated dashboards offer updates in real-time, enabling important choices to be made with the latest information available, which reduces delays common with manual reporting systems. The systems are very easy to use, which means even staff who don't know coding can work with data, by exploring the interactive parts, leading to wider data literacy throughout any given organization. Automated systems often come with ways of tracking the effectiveness of dashboards, with information on who uses them and how often. This helps with improving dashboards continuously. Integration into various different source systems, is also a crucial feature, allowing these systems to automatically receive data from any source, creating a simplified and single view. The technology powering these systems makes use of machine learning that attempts to do things automatically with less human oversight. By improving it output using the usage and feedback, a cycle of continual improvement is promoted. It is quite interesting how changes in reporting times, can lead to shifts in project management, so teams might plan proactively instead of reacting, when developing new strategies using data.
7 Ways AI-Enhanced Self-Service BI Tools Are Transforming Data Analysis in 2025 - Real-Time Pattern Detection Alerts Users to Data Anomalies Before Problems Occur
In 2025, the capacity to detect patterns in real-time is emerging as a vital component of self-service BI tools. This feature actively notifies users about unusual data behaviors, giving them a chance to address potential problems before they become serious. Utilizing sophisticated algorithms, these tools constantly analyze data as it comes in, enabling a more forward-thinking approach to data handling within organizations. Such immediate notifications about data changes not only prevent possible disruptions, but they also allow people to act on up-to-date information. With continuous progress in artificial intelligence, the precision of these systems in identifying abnormal data is likely to improve, making them essential for companies looking to keep their data reliable and operations running smoothly. Nevertheless, although these improvements make data use simpler, they also create some worries about depending too much on automatic tools, possibly undermining the significance of individual supervision and thoughtful examination of data.
Real-time pattern detection employs sophisticated algorithms designed to analyze extensive data streams with speed. The goal is to spot anomalies in data, happening live, instead of only afterwards when things might already be critical. These systems are designed to discern between normal data shifts and meaningful outliers. This capability reduces the occurrence of false alerts that often plague less advanced methods that result in distractions. By utilizing predictive modeling and clustering, these alerts are designed to not only be reactive, but they can now forecast potential issues by learning from historical data trends. The implementation of ensemble learning, combining several models to ensure accuracy, is also common now, which is better than relying on single analytic approach. Industries like finance and health care see particular use where catching irregular events quickly can help prevent fraud or prevent health issues worsening. User-friendly interfaces are available to display alerts, no expert knowledge needed. This approach allows those in non-technical roles to receive important alerts and issues. As they are fed with new data the machine learning models constantly update, adapting and changing. The real effectiveness depends very heavily on the quality of data being feed into the system. Bad quality data can lead to incorrect alerts, therefore emphasizing the critical nature of data management. Organizations using these systems save resources by focussing efforts on genuine anomalies and resolving issues early. Real-time anomaly detection technologies can provide sophisticated insights when integrated with business intelligence software. A view of organizational performance is enhanced allowing for identification of potential risk factors.
7 Ways AI-Enhanced Self-Service BI Tools Are Transforming Data Analysis in 2025 - Predictive Analytics Models Now Self-Train on Historical Company Data
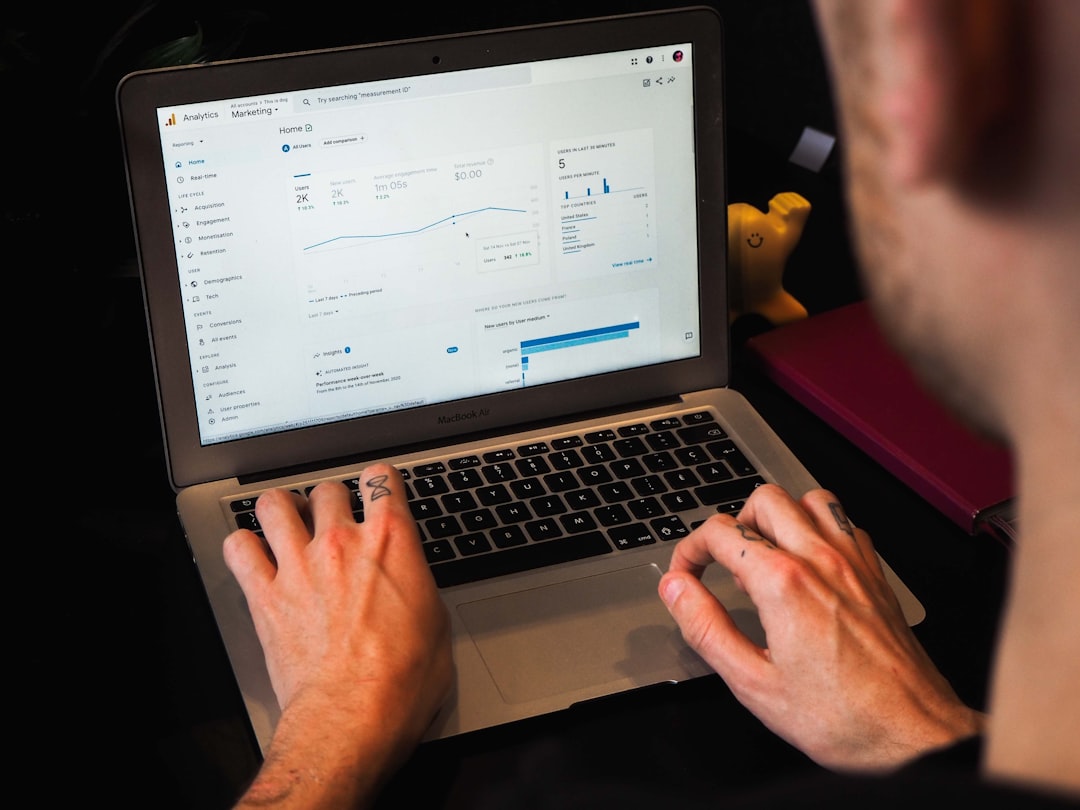
Predictive analytics models are now capable of self-training using a company's historical data. This allows organizations to analyze their past to better predict future trends. The success of this approach depends on the power of the analytics software to process large datasets and reveal useful patterns. With the inclusion of AI and machine learning, these techniques have become more accessible through self-service business intelligence (BI) tools, often needing little technical expertise from the user. However, the effectiveness of these predictive models is highly dependent on the accuracy and quality of the data they are trained on, making the governance of data of critical importance for those using such systems. As these self-training systems are more widely adopted, data reliability and management become crucial considerations for organizational decision-making.
Predictive analytics models are now designed to self-train using a company's historical data, refining themselves without explicit instruction or constant manual tuning, allowing organizations to focus their energy on strategic decisions. This adaptive learning technique enables the models to adjust their internal parameters as they process new data, enabling a continuously evolving and more nuanced picture of trends. It is interesting to see how these models are starting to take in a larger view of operations by incorporating diverse datasets from sales records, customer interactions to even the often ignored social media metrics; this means, organisations can be more agile when acting on potential advantages that otherwise might be overlooked. The speed at which these predictive models can now processes data is impressive. Their ability to immediately turn historical data into actionable insights helps companies significantly accelerate their decision making processes. The problem of data overfitting is another challenge, however, the self-training algorithms seem to be actively working to minimise the issue and their ongoing engagement with new data means the models retain their predictive accuracy. These systems now automatically make intelligent choices when selecting the most appropriate model depending on the incoming information, taking away the guesswork for what traditionally required expertise. We're also now seeing how these models have the ability to provide insights across different areas of a business, which provides a much more integrated view, rather than working in siloes, which might be leading to novel business strategies. Furthermore, data visualizations are being enhanced too, making it more efficient for stakeholders to grasp complicated information via easy to understand graphics. However, it is now the user who drives the process, by choosing specific parts of the data for model training which introduces some level of customisation and also makes people more actively involved in the analysis process. These models learning from historical data also bring about critical ethical challenges, since old data contains bias from previous decision making processes. Data bias and oversight are crucial to ensure these tools are not simply perpetuating the inequalities present in historical data.
7 Ways AI-Enhanced Self-Service BI Tools Are Transforming Data Analysis in 2025 - Voice-Controlled Analytics Interface Makes Data Exploration Hands-Free
Voice-controlled analytics interfaces are changing the way people interact with data, offering a hands-free approach to exploring information and greatly simplifying analytical tasks. These systems enable users to engage with data using spoken commands, making robust tools available to a broader audience, including those who may lack technical skills. This shift not only makes data queries more direct, but can also promote collaboration and improve decision-making speeds. However, as convenient as voice-based systems are, some worry about accuracy and a possible overdependence on automatic processes. This means it remains very important that organizations critically oversee the use of these technologies. As AI continues to advance in data analysis, understanding its limits remains important if these changes in data analytics are to be used effectively.
Voice-controlled data analytics is beginning to enable hands-free interaction with information, allowing users to explore data just by using their voices, potentially leading to more efficient workflows. These systems rely on highly sensitive speech recognition to handle data questions in real-time. This approach provides instant feedback when exploring complex data, allowing professionals in areas like finance or health to rapidly get the results they need. The possibility for conversational interactions with data may improve user experience but also demands a level of sophistication in algorithms capable of maintaining the context across multiple follow up questions. Some systems are already combining speech input with visual prompts to manage complex data analysis remotely or without heavy reliance on screens. These systems potentially improve as they get used more often by a user, learning individual patterns and preferences, therefore leading to personalized improvements. Reducing typical data handling errors, like typos is a major benefit as these voice commands replace manual data entries. There is potential in future for integration with IOT devices to directly incorporate external data feeds into the analysis. This hands-free ability opens up data analysis for those with accessibility issues who might struggle with traditional software interfaces. This tech allows users to follow up with nuanced questions within the flow of a conversation, deepening insights into the data, something that is clunky in traditional systems. Despite improvements, there can still be issues with processing non standard or unusual language, including jargon, colloquialisms or strong accents which highlight the continued need for work in natural language processing.
7 Ways AI-Enhanced Self-Service BI Tools Are Transforming Data Analysis in 2025 - Automated Data Cleaning Reduces Preparation Time by 70%
Automated data cleaning has emerged as a transformative force in data analysis, capable of slashing preparation time by up to 70%. This stark reduction in time spent on tasks traditionally burdened by manual inputs allows analysts to redirect their focus towards more strategic activities, such as trend identification and predictive modeling. With minimal human oversight required, automated processes enhance data accuracy and reliability over time, ensuring that organizations can depend on quality insights for decision-making. As this technology evolves, its integration with other intelligent tools creates opportunities for real-time monitoring and anomaly detection, further optimizing the data management landscape. Nonetheless, while automation reduces the burden, there persists a need for human expertise to contextualize and interpret data effectively.
Data preparation usually accounts for a surprising amount of time in any data project; so much so that it can eat up nearly 80% of a project's schedule. That's why the idea of cutting that time by 70% with automated cleaning is such a big deal. These automated systems use smart algorithms to identify bad entries, duplications and unusual data points; reducing human errors that come from manual checks by as much as 90% in some cases. Unlike humans, they also have no problem handling vast amounts of data – petabytes even – which is very important as datasets keep getting bigger. They integrate quite easily with multiple data sources, allowing continuous data cleaning directly at source, avoiding old, manual processes. By spending less time doing data cleaning, organizations save money too, about a quarter of the usual data handling costs, which is not a small amount. Some systems even fix missing information using other sources. So you get a dataset which is both cleaned and more informative, which improves later analysis. Many of these advanced systems check data in real-time, which ensures data remains consistent and good as new sources come in. This kind of constant watch also makes sure that data regulations are followed, lowering the chances of legal problems. Users from all levels can start analyzing the data, as automated cleaning simplifies the process, shifting reliance away from data teams, democratizing access to important insights. Perhaps most importantly, these systems keep improving by using machine learning, becoming better at finding and fixing issues as they keep working through more data.
7 Ways AI-Enhanced Self-Service BI Tools Are Transforming Data Analysis in 2025 - Cross-Platform Integration Enables Unified Analysis Across All Business Tools
Cross-platform integration is becoming essential as businesses grapple with data spread across different systems. Combining information from various sources lets organizations analyze data together, improving decision-making and overall efficiency. AI and machine learning are now vital in managing these complex data integrations, allowing businesses to use data assets more effectively. This approach minimizes data silos, providing stakeholders with comprehensive insights to help inform strategy. However, the heavy reliance on these systems raises questions about data quality and management, making careful oversight key to achieving the desired outcomes.
Cross-platform integration now focuses on connecting different business software, letting companies pull data from different systems without too much manual work. This is designed to reduce data errors and the time wasted on making data compatible. This unified approach gives a broader view of business activities, by bringing together information from many departments into one central view. This is hoped to help decision-makers to react faster to new findings and also help create a more data-based organization using all its collective resources. By needing fewer specialist tools, companies are looking to cut down costs and use their resources more intelligently, leading to more streamlined processes. When different systems are linked together, the aim is to keep data more reliable by using standard data formats, as well as checks and validations, meaning that insights can be more trustworthy. Furthermore, different teams can work at the same time and quickly change the project direction depending on the latest results, because they are all looking at the same live data. This integrated approach also promises to help scale data capabilities, because adding new tools or changing business needs might be more easily implemented compared to less interconnected systems. Automatic reports can also now be made using the latest data, which means important stakeholders might not need manual data compilations to receive regular updates. However, concerns around data governance and security are becoming very important in this type of integration because protocols are necessary to manage who has access to confidential data. The use of APIs helps facilitate this seamless flow of data between different systems, which is very important for companies who now rely on multiple software solutions for their day to day activities.
Revolutionize your business strategy with AI-powered innovation consulting. Unlock your company's full potential and stay ahead of the competition. (Get started for free)
More Posts from innovatewise.tech: